En este artículo, vamos a hacer un proyecto de Python que use OpenCV y Mediapipe para ver el gesto de la mano y, en consecuencia, establecer el brillo del sistema en un rango de 0 a 100.
Hemos utilizado un módulo HandTracking que rastrea todos los puntos de la mano y detecta los puntos de referencia de la mano, calcula la distancia entre la punta del pulgar y la punta del dedo índice y mapea la distancia entre la punta del pulgar y la punta del dedo índice con el rango de brillo.
Bibliotecas requeridas
- Mediapipe: es el marco de código abierto de Google, utilizado para el procesamiento de medios. Es multiplataforma o podemos decir que es amigable con la plataforma. Puede ejecutarse en Android, iOS y la web, eso es lo que significa multiplataforma, para ejecutarse en todas partes.
pip install mediapipe
- OpenCV : Es una biblioteca de Python que está diseñada para resolver problemas de visión por computadora. OpenCV admite una amplia variedad de lenguajes de programación como C++, Python, Java, etc. Compatibilidad con múltiples plataformas, incluidas Windows, Linux y MacOS.
pip install opencv-python
- Screen-Brightness-Control: Es una herramienta de Python para controlar el brillo de su monitor. Admite Windows y la mayoría de las versiones de Linux.
pip install screen-brightness-control
- Numpy : es un paquete de procesamiento de arrays de propósito general. Proporciona un objeto de array multidimensional de alto rendimiento y herramientas para trabajar con estas arrays. Es el paquete fundamental para la computación científica con Python.
pip install numpy
Implementación paso a paso
Paso 1: importa todas las bibliotecas requeridas
Python3
# Importing Libraries import cv2 import mediapipe as mp from math import hypot import screen_brightness_control as sbc import numpy as np
Paso 2: Inicializar el modelo Hands
Python3
# Initializing the Model mpHands = mp.solutions.hands hands = mpHands.Hands( static_image_mode=False, model_complexity=1, min_detection_confidence=0.75, min_tracking_confidence=0.75, max_num_hands=2) Draw = mp.solutions.drawing_utils
Veamos los parámetros para el modelo de manos:
Manos (static_image_mode=False, model_complexity=1 min_detection_confidence=0.75, min_tracking_confidence=0.75, max_num_hands=2)
Dónde:
- static_image_mode: se usa para especificar si la imagen de entrada debe ser una imagen estática o una transmisión de video. El valor predeterminado es Falso.
- model_complexity: Complejidad del modelo de punto de referencia de la mano: 0 o 1. La precisión del punto de referencia, así como la latencia de inferencia, generalmente aumentan con la complejidad del modelo. Predeterminado a 1.
- min_detection_confidence: Se utiliza para especificar el valor mínimo de confianza con el que se debe considerar exitosa la detección del modelo de detección de personas. Puede especificar un valor en [0.0,1.0]. El valor predeterminado es 0,5.
- min_tracking_confidence: Se utiliza para especificar el valor mínimo de confianza con el que se debe considerar exitosa la detección del modelo de seguimiento de hitos. Puede especificar un valor en [0.0,1.0]. El valor predeterminado es 0,5.
- max_num_hands: Número máximo de manos a detectar. Por defecto es 2.
Paso 3: Procese la imagen y aplique brillo en función de la distancia entre la punta del dedo pulgar y el índice
Capture los cuadros continuamente desde la cámara usando OpenCV y convierta la imagen BGR en una imagen RGB y haga predicciones usando el modelo de manos inicializado. La predicción realizada por el modelo se guarda en la variable de resultados desde la que podemos acceder a los puntos de referencia mediante results.multi_hand_landmarks y, si hay manos presentes en el cuadro, detectar los puntos de referencia de las manos y luego calcular la distancia entre la punta del pulgar y la punta del dedo índice. Mapee la distancia de la punta del pulgar y la punta del dedo índice con el rango de brillo, es decir, según la distancia entre ellos, cambiará el brillo del sistema.
Python3
# Start capturing video from webcam cap = cv2.VideoCapture(0) while True: # Read video frame by frame _,frame = cap.read() #Flip image frame=cv2.flip(frame,1) # Convert BGR image to RGB image frameRGB = cv2.cvtColor(frame,cv2.COLOR_BGR2RGB) # Process the RGB image Process = hands.process(frameRGB) landmarkList = [] # if hands are present in image(frame) if Process.multi_hand_landmarks: # detect handmarks for handlm in Process.multi_hand_landmarks: for _id,landmarks in enumerate(handlm.landmark): # store height and width of image height,width,color_channels = frame.shape # calculate and append x, y coordinates # of handmarks from image(frame) to lmList x,y = int(landmarks.x*width),int(landmarks.y*height) landmarkList.append([_id,x,y]) # draw Landmarks Draw.draw_landmarks(frame,handlm,mpHands.HAND_CONNECTIONS) # If landmarks list is not empty if landmarkList != []: # store x,y coordinates of (tip of) thumb x_1,y_1 = landmarkList[4][1],landmarkList[4][2] # store x,y coordinates of (tip of) index finger x_2,y_2 = landmarkList[8][1],landmarkList[8][2] # draw circle on thumb and index finger tip cv2.circle(frame,(x_1,y_1),7,(0,255,0),cv2.FILLED) cv2.circle(frame,(x_2,y_2),7,(0,255,0),cv2.FILLED) # draw line from tip of thumb to tip of index finger cv2.line(frame,(x_1,y_1),(x_2,y_2),(0,255,0),3) # calculate square root of the sum # of squares of the specified arguments. L = hypot(x_2-x_1,y_2-y_1) # 1-D linear interpolant to a function # with given discrete data points # (Hand range 15 - 220, Brightness range 0 - 100), # evaluated at length. b_level = np.interp(L,[15,220],[0,100]) # set brightness sbc.set_brightness(int(b_level)) # Display Video and when 'q' is entered, # destroy the window cv2.imshow('Image', frame) if cv2.waitKey(1) & 0xff == ord('q'): break
A continuación se muestra la implementación completa:
Python3
# Importing Libraries import cv2 import mediapipe as mp from math import hypot import screen_brightness_control as sbc import numpy as np # Initializing the Model mpHands = mp.solutions.hands hands = mpHands.Hands( static_image_mode=False, model_complexity=1, min_detection_confidence=0.75, min_tracking_confidence=0.75, max_num_hands=2) Draw = mp.solutions.drawing_utils # Start capturing video from webcam cap = cv2.VideoCapture(0) while True: # Read video frame by frame _, frame = cap.read() # Flip image frame = cv2.flip(frame, 1) # Convert BGR image to RGB image frameRGB = cv2.cvtColor(frame, cv2.COLOR_BGR2RGB) # Process the RGB image Process = hands.process(frameRGB) landmarkList = [] # if hands are present in image(frame) if Process.multi_hand_landmarks: # detect handmarks for handlm in Process.multi_hand_landmarks: for _id, landmarks in enumerate(handlm.landmark): # store height and width of image height, width, color_channels = frame.shape # calculate and append x, y coordinates # of handmarks from image(frame) to lmList x, y = int(landmarks.x*width), int(landmarks.y*height) landmarkList.append([_id, x, y]) # draw Landmarks Draw.draw_landmarks(frame, handlm, mpHands.HAND_CONNECTIONS) # If landmarks list is not empty if landmarkList != []: # store x,y coordinates of (tip of) thumb x_1, y_1 = landmarkList[4][1], landmarkList[4][2] # store x,y coordinates of (tip of) index finger x_2, y_2 = landmarkList[8][1], landmarkList[8][2] # draw circle on thumb and index finger tip cv2.circle(frame, (x_1, y_1), 7, (0, 255, 0), cv2.FILLED) cv2.circle(frame, (x_2, y_2), 7, (0, 255, 0), cv2.FILLED) # draw line from tip of thumb to tip of index finger cv2.line(frame, (x_1, y_1), (x_2, y_2), (0, 255, 0), 3) # calculate square root of the sum of # squares of the specified arguments. L = hypot(x_2-x_1, y_2-y_1) # 1-D linear interpolant to a function # with given discrete data points # (Hand range 15 - 220, Brightness # range 0 - 100), evaluated at length. b_level = np.interp(L, [15, 220], [0, 100]) # set brightness sbc.set_brightness(int(b_level)) # Display Video and when 'q' is entered, destroy # the window cv2.imshow('Image', frame) if cv2.waitKey(1) & 0xff == ord('q'): break
Producción:
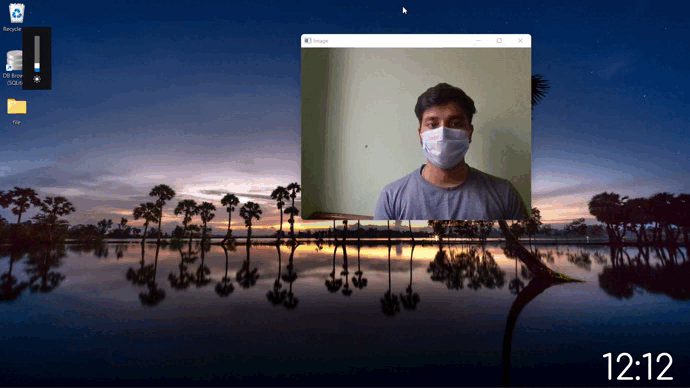